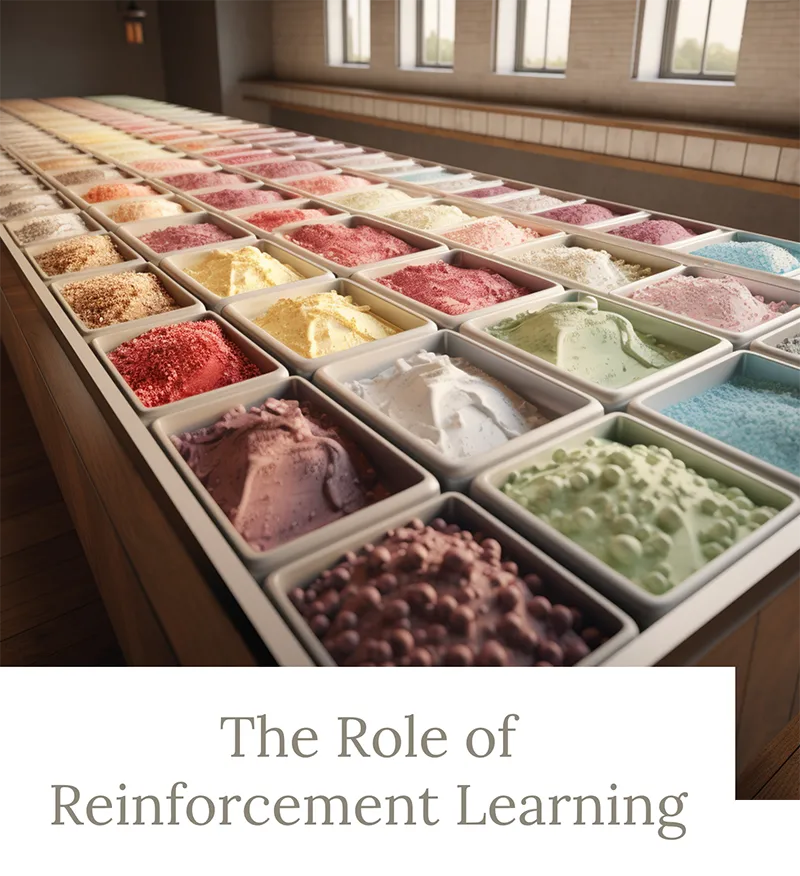
Cognitive science can’t settle questions of right and wrong. But it can set the table for theories of rational decision-making and ethics. And the one thing we know for sure about how we think is that, at the most fundamental level, our brains are connection systems that are changed by experience.This matters; transformational experiences and self-altering decisions exist because of the way we think. Our minds change. They change in the shallow sense of changing what we remember, the facts we know or the beliefs we hold…but they also change in a much deeper sense. Experience changes, in fundamental ways, how we think and who we are. That means that a rational decision-maker must consider how experience will change them. This also means that, since we can to some extent select what kind of experiences to have, we have some control over how we change.
But though learning is at the heart of cognition and though we are exceptional learners, the basic architecture of cognition creates both challenges for and limitations on the decision-maker. The nature of connectionist learning accounts for many of the ways in which people tend to be irrational about beliefs and decisions. These limitations on rationality are, however, only a part of the story. Humans learn, but our brains have evolved to focus and control the extent of cognitive change based on aspects of experience. Understanding how this works is essential to an appreciation of what kinds of experience make for effective (or disastrous) cognitive change.
Connection systems typically work by tuning the network based on training data. Each datum given the network comes with a label – telling the network the right answer. Based on the label, the network adjusts its weights and connections to produce a better answer next time. Do this millions of times, and a connection system can get incredibly good at very complex actions. But, of course, the world doesn’t come with training data – so how do our minds learn? It turns out that our brains contain layers of connection systems each of which generates data for the layer above it and predictions based on the layer(s) below it. This allows us to, in effect, learn as we go.
The brain’s use of prediction and hierarchical connection systems to generate training data is remarkable and helps explain how we can reliably track to reality and make sense of the world around us. But from a decision-making perspective, this part of cognition is table-stakes. We take for granted our ability to understand and predict basic events in our environment. Recognizing dogs or catching baseballs in flight is a lot harder than it seems, but it isn’t the sort of thinking we fret over. In the decision-makers world, prediction and understanding are tools for decision-making about action. And in the world of action, reinforcement learning is the key.
It was only a few years ago that a Google-built computer program beat the world-champion at the game of Go. Similar systems have mastered the complex world navigation and tactics of massive multi-player online and computer games. These programs are connection systems, but they don’t use traditional supervised training methods (giving the right answer along with an input). In games like Go, the complexity of the decision tree is far too high to capture with a bunch of board positions and labels (there are just too many options at any given point). Instead, these programs used reinforcement learning to train the connection system. The network trains to an outcome (“game points” or “win”) not a label (“dog” or “no dog”).
This may seem an obvious, even trivial, substitution but replacing labels with outcomes creates significant new learning challenges. As a discipline, reinforcement learning is about finding strategies that create optimal outcomes (maximization) in situations with a large or infinite number of possible choices. Situations like life. We aren’t here concerned with decision-theory and preference optimization (which get plenty of attention across multiple disciplines), but even basic preference optimization decisions are surprisingly complex in a world where we must learn what we like. Because a decision-maker’s preference set is limited by prior experience, it’s often necessary to make choices about whether to optimize to known factors or explore potential new alternatives.
Here’s a simple example. Say you go into an ice-cream shop with 33 flavors once a month. You’ve tried five flavors of ice-cream in your life, and you know you like Strawberry and Mango the best and Vanilla and Coffee the least with Chocolate playing Mr. In-Between. How often, when you go into the store, should you try a new flavor? Every time? If you do that, it will be a long time till you’ve tried them all and can have Strawberry again. Never? That seems wrong too – what if there are flavors out there that you’d like MUCH better even than Strawberry? The right strategy will likely depend on how definitive your preference for Strawberry is after trying five flavors, how many choices are available to you, and how often you get ice cream. There is no obvious answer to the question of what to have next or how often to try a new flavor – and yet this is about the simplest problem in choice and decision-making imaginable.
Reinforcement learning methods solve problems like this – especially in situations where there are a LOT of choices. What makes reinforcement learning particularly germane here is that it’s an adaptive learning technique. All reinforcement learning strategies use prior experience to improve future outcomes and part of what they do is suggest strategies for selecting future experiences to optimize learning not optimize known preferences. This isn’t quite self-altering decision-making (based on transformative experience) since all reinforcement learning is still about optimization, but it’s in the same ballpark.
In the computer world, reinforcement learning is used to drive connection systems because they allow those systems to be much less structured and more general. The Google system[1] that became a Go master was able, with NO additional programming, to become almost unbeatable at chess too. A computer connection system can optimize its internal network using the score or outcome of almost any game.[2]
People, of course, do not have the option of choosing between a connection system or algorithmic architecture. Since connection systems are what the brain is made of, it’s important that connection systems can be trained – not just with labels – but with outcomes. Because while understanding the world is an essential tool for a decision-maker, outcomes are what drive choice. Preferences, after all, are outcomes. We make decisions that drive actions that satisfy preferences. When we get what we want, both the satisfaction of the desire and of accomplishing an action contribute positive reinforcement. As babies and children, we repeat actions that give us positive reinforcement. Food, comfort, attention. That’s how our brains work.
At the same time, negative reinforcements create their own kind of learning. Failure, pain and discomfort create guidelines within which learning takes place. For a piano student, the most effective learning reinforcement is positive – hearing the music creates its own immediate pleasure. One of the reasons people find it easier to learn skills than change dispositions is that most skills create direct positive reinforcement when done well. Praise from a teacher may provide additional reinforcement, and applause or parental pride even more. Yet building skills is invariably hard, toilsome work. In the short run, there are nearly always other actions with higher immediate preference satisfaction values. Negative reinforcement via criticism, punishment or failure helps enforce the discipline necessary to get to the positive outcomes.
Nearly all learning involves both positive and negative reinforcement and the optimal balance between them isn’t carved in stone. Setting aside differences in individual psychology, the degree to which the learning activity generates internal satisfactions will often determine the best mix of positive and negative reinforcement or the nature of the reinforcement used. One of the reasons a tone-deaf student will struggle to learn the piano is that the activity provides no positive reinforcement. In theory, a person could learn to play mechanically and to hone specific motor movements based on praise from an instructor or a score from an AI. Absent the reward of hearing the success, however, learning is extremely difficult.
Just as categorization requires labels (correct answers) to drive learning, decision-makers need a measure of success to tune action. People don’t have to search around for those reinforcement signals, as embodied beings we generate them constantly. For every action (and every inaction), the body feeds back signals of pleasure or pain, satisfaction, or discomfort. These signals cannot be ignored, and they always drive learning.
At the most basic level, brains learn BECAUSE they are embodied.
This isn’t to denigrate natural curiosity or understanding for understanding’s sake. Humans are general-purpose learners. We can’t help but learn. We can’t stop. But we are not just thinkers. We are doers. And we are listeners. Listeners to what our world and our bodies are saying. We are born caring and at that, too, we never stop.
As a baby, most of the feedback we get involves the basics of embodiment. Yet the utter helplessness of the human baby ensures that even at the youngest ages, we are deeply attuned to people. Humans are more social than ants. We are born to recognize faces. To smile. To laugh. To be tickled. When a baby learns to move the muscles in its face into a smile, the feedback is off-the-charts.
The nature of preferences and reinforcements change as we mature. But feedback and reinforcement remain critical aspects of learning and feedback is inherently social. Few of us can learn anything complex or hard without external positive feedback on success. This isn’t the same thing as a machine learning requirement to have “training” data that contains the correct answer. Yes, many computer prediction systems need to have the right answer flagged so that the network can tune itself. We’ve already seen how the brain uses its own system arranged in multiple hierarchies to generate predictions which are then confirmed or rejected to effectively self-generate that kind of training data. But while computer systems train simply because that’s what they are designed to do, human cognition takes advantage of positive feedback not only to flag good answers, but to weight attention, understanding and action opportunities based on the strength and nature of that feedback.
For humans, having training data isn’t enough. We need a reason to process it. Preference sets drive learning, and few people can learn effectively in the absence of feedback loops reinforcing the process.
We learn to learn. And we learn because we have preferences. Preferences serve an essential function in making learning happen. The brain’s dependency on experience is also, though less completely, a dependency on reinforcement. It’s not impossible to learn without reinforcement, it’s just very difficult. And this dependency has deep implications for transformational choice. Our reliance on social feedback and external reinforcement means that we are nearly always reliant on our culture to help us achieve whatever transformational outcomes are desired.
Understanding how the brain changes (experience) and what it needs to drive learning (reinforcement) helps explain why so many of our efforts to change who we are turn out to be ineffectual. You can tell yourself to be nicer ten times every day and, without either experience or reinforcement, those admonishments will achieve nothing. Nobody expects to become an accomplished pianist by repeatedly telling themselves to be a better pianist!
Practical educators have always understood the essential role of habit, repetition and reward in creating cognitive structure. But there has been a tendency, in the ethical realm, to denigrate acts designed to mimic dispositions you do not feel. Given our cognitive architecture, this is a mistake. To become kind, you must act kind and you must find and select environments (and actions) that reward kindness. Aristotle put it this way: “For the things we have to learn before we can do them, we learn by doing them, e.g. men become builders by building and lyre players by playing the lyre, so too we become just by doing just acts, temperate by doing temperate acts, brave by doing brave acts.” But the doing of the act isn’t enough. Repetition only breeds structure when accompanies by reinforcement. We only learn when we have a reason.
When it comes to transformative experience and self-altering decisions, the central role of reinforcement learning in cognition underscores the importance of finding micro-cultures and situations that provide the right kind of reinforcement. Good feedback loops are essential to cognitive training and if we’re choosing experiences based on their transformative nature, we need to think about how and where reinforcement will come from. In practice, it will often be a matter of who you have the experience with. The teacher you have, the mentor you choose, the friends you hang out with. They provide, for good or ill, much of the feedback you get. And just as you can choose some parts of what you experience, you also have some level of choice about who you experience it with. It’s an aspect of choice we frequently ignore, but it matters enormously.
Cognitive science will never and can never tell us what kind of person to be. But it can and does suggest that some learning strategies are far more natural and efficacious than others and that some do not work at all. The fundamental guardrails to cognitive change are defined by the basic and uncontroversial structure of the brain as a connection system driven by prediction and reinforcement learning.
[1] Or at least it’s successor.
[2] Reinforcement-learning computer-based connection systems are unbeatable at games like chess and Go because of their raw speed not the efficiency of their learning. These computer systems can play thousands of games in the time it takes a human to play one. In a month, they can play more games than a human player will in a lifetime. Not every experience can be digitally simulated and sped up 10,000x, but for any experience that can be, a human will never again be able to compete against a computer. We’re doing the same kind of learning, but we can’t experience nearly as much.